Generative artificial intelligence (AI) tools, including large language models, image synthesis engines, and autonomous decision systems, are transforming operations across the financial sector. Institutions are adopting these tools to automate processes, accelerate decision-making, and enhance customer experience. However, unlike traditional software, generative models introduce unpredictable behavior, opaque reasoning, and complex data exposure risks. Without formal oversight, these systems can compromise fairness, security, and regulatory compliance.
To address these challenges, the National Institute of Standards and Technology (NIST), Microsoft Security, and the U.S. Department of the Treasury have issued frameworks and policy recommendations to manage AI risks through improved transparency, governance, lifecycle monitoring, and regulatory coordination.
NETBankAudit leverages these resources to help financial institutions evaluate generative AI risks and strengthen internal controls. Our facilitation process includes:
- A workbook of all reviewed controls and associated risks
- A management report summarizing findings and recommendations
- A policy template for safe and compliant use of generative AI
For more information on this service, request a proposal. The following article introduces seven key action areas based on guidance from NIST, Microsoft, and the U.S. Department of the Treasury.
Key Frameworks Informing Generative AI Risk
Before diving into the seven core action areas, it’s important to understand the foundational guidance shaping current best practices. NETBankAudit draws from three authoritative sources—each offering a distinct lens on AI risk management in the financial sector.
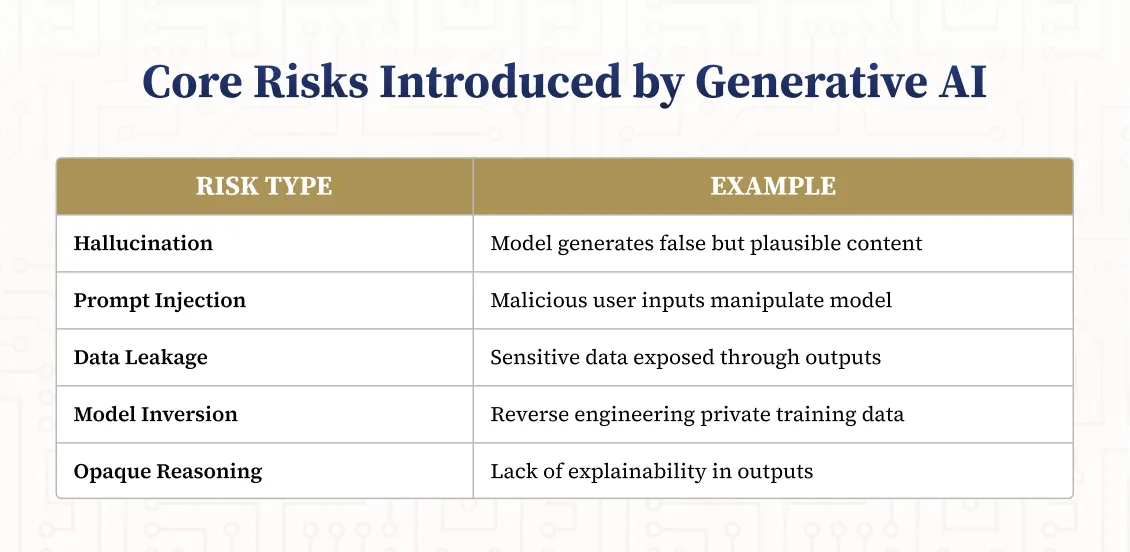
NIST AI Risk Management Framework (AI RMF)
The NIST AI Risk Management Framework (AI RMF) provides a structured approach for identifying, assessing, and mitigating AI-related risks across the full lifecycle of development and deployment. Its four core functions (Govern, Map, Measure, and Manage) establish a foundation for building trustworthy AI systems that prioritize security, explainability, fairness, and reliability. In July 2024, NIST released the Generative AI Profile (NIST AI 600-1), a companion resource that offers specific recommendations for managing risks unique to generative AI technologies, such as hallucination, data leakage, and prompt injection. This profile enhances the RMF by aligning it with emerging use cases and regulatory expectations in sectors like financial services.
Microsoft Secure Future Initiative
Microsoft’s AI Security and Governance Guidance outlines practical safeguards for building secure, explainable, and compliant AI systems. Microsoft places emphasis on threat modeling, lifecycle governance, vendor oversight, and incident response in the context of generative AI tools, including large language models and image generators. Their playbooks are particularly useful for understanding implementation-level risks and enterprise security posture.
Treasury Department AI Guidance (December 2024)
In December 2024, the U.S. Department of the Treasury published its report on Artificial Intelligence in Financial Services, summarizing responses from over 100 stakeholders and outlining policy considerations, risks, and recommendations for AI deployment. Unlike earlier general guidance, this report focuses specifically on AI use in financial institutions, including credit underwriting, fraud detection, risk management, and customer service.
Treasury’s key observations and recommendations include:
- Aligning AI definitions to reduce regulatory fragmentation
- Enhancing data privacy, quality, and security standards, especially in third-party and generative AI contexts
- Requiring explainability and fairness across customer-facing models
- Evaluating existing compliance frameworks to ensure they sufficiently cover AI-specific risks (e.g., discrimination, hallucination, systemic concentration)
- Promoting public-private partnerships and international regulatory alignment
Addressing the growing third-party reliance risk, particularly for small and mid-sized institutions
This guidance, while not legally binding, signals Treasury’s intent to support interagency coordination, define supervisory expectations, and close regulatory gaps. It complements technical frameworks like NIST’s AI RMF and Microsoft’s secure lifecycle governance model.
7 Action Areas for Managing Generative AI Risk
Effective oversight of generative AI depends on strong governance across multiple operational domains. Drawing from NIST, Microsoft, and the U.S. Department of the Treasury, this section identifies seven key areas for institutional focus: AI policy, threat environment, vendor oversight, data controls, AI model management, system resiliency, and compliance. Each area reflects overlapping priorities across these frameworks, offering financial institutions a path to align internal controls with evolving regulatory expectations and industry best practices.
1. AI Policy
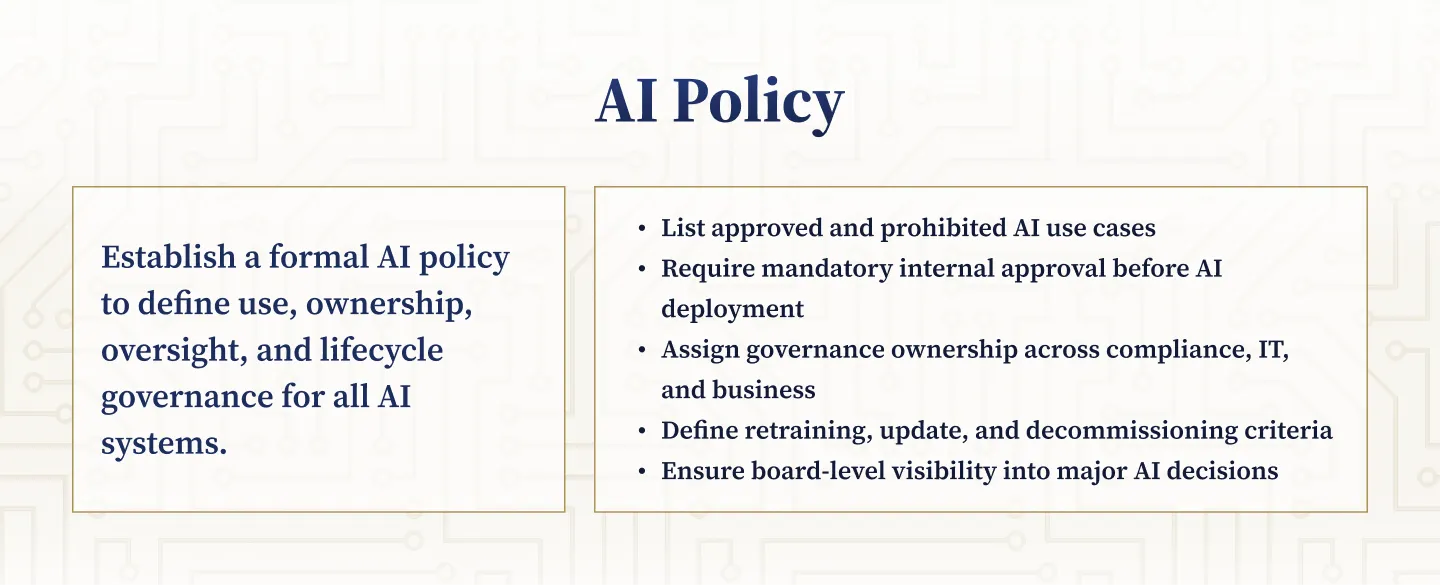
Aligning AI Use with Institutional Governance
Establishing a formal AI policy is foundational to responsible deployment and governance. NIST’s “Govern” function emphasizes the need for institutions to define and document how AI systems are authorized, monitored, and maintained. Microsoft reinforces this in its AI governance guidance, calling for cross-functional accountability and clear lifecycle policies. The U.S. Department of the Treasury highlights policy development as a key area where financial institutions must reduce ambiguity, ensure consistency across business units, and clarify roles for board-level and executive oversight. Treasury also notes that gaps in governance often leave smaller institutions especially vulnerable to third-party risk and inconsistent compliance practices.
What an Effective AI Policy Should Cover
- A defined list of approved and prohibited AI use cases, with criteria for periodic updates as technology evolves
- Mandatory internal approvals before any AI system is deployed, including pilot projects
- Assignment of clear ownership for AI governance across compliance, information security, and business operations
- Criteria for reviewing, retraining, or decommissioning AI systems based on performance or risk exposure
- Board-level visibility into major AI-related decisions, especially those affecting regulatory compliance or customer outcomes
For institutions without a formal AI policy in place, NIST’s AI Risk Management Framework and Microsoft’s governance templates offer publicly available structures to build from. Treasury’s 2024 report further supports this approach by encouraging proactive alignment with supervisory expectations and cross-agency definitions of AI system scope.
2. Threat Environment
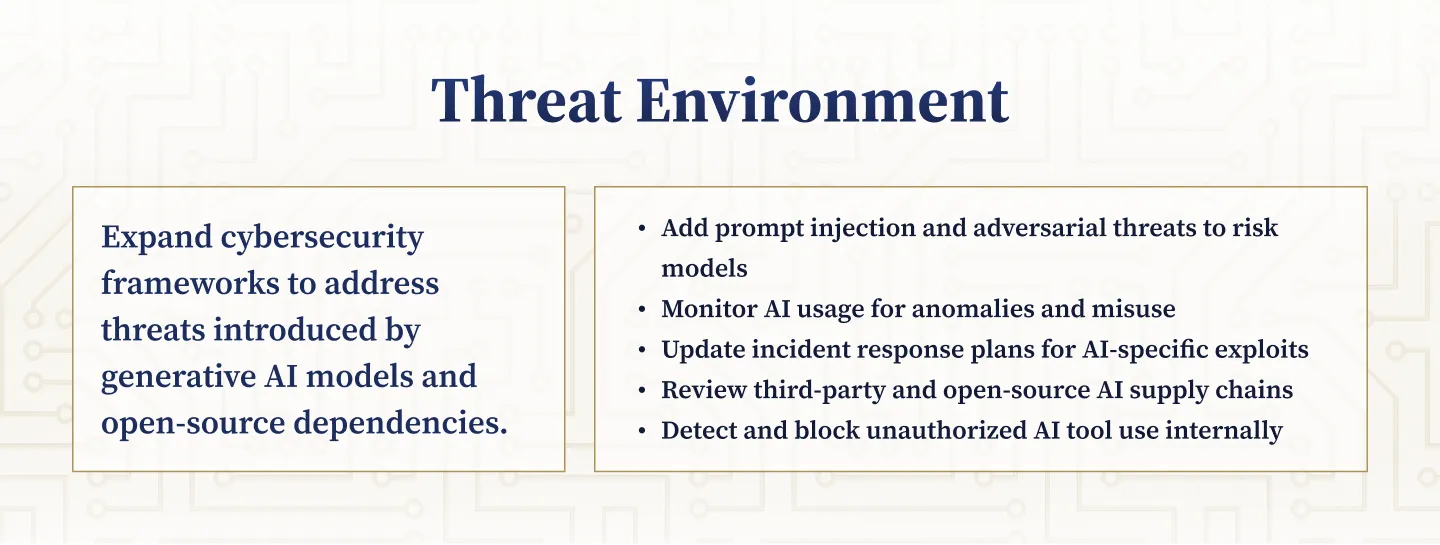
Recognizing New Threats Introduced by Generative Models
Generative AI introduces novel cybersecurity risks that extend beyond traditional controls. Threats such as prompt injection, adversarial prompts, data leakage, model inversion, and training data poisoning require institutions to rethink their incident response strategies. NIST’s “Measure” and “Manage” functions emphasize the need for continuous threat assessment, detection capabilities, and response protocols tailored to AI-specific vulnerabilities. Microsoft’s guidance expands on this by urging organizations to integrate AI threats into their overall cyber defense strategy.
The U.S. Department of the Treasury calls on institutions to actively update risk models to reflect generative AI misuse scenarios. The 2024 report notes that financial firms face elevated exposure due to open-source toolchains, third-party dependencies, and the complexity of auditing AI-generated decisions. Treasury also stresses that boards and executives must consider these threats as part of broader operational risk.
How Institutions Can Expand Threat Coverage
- Include AI-specific scenarios in tabletop exercises and threat modeling
- Log prompt inputs, outputs, and system usage for anomaly detection and misuse
- Review the full AI supply chain, including third-party and open-source models
- Update incident response plans to account for AI-specific exploits like prompt injection or hallucinated content
- Restrict unauthorized internal use of generative AI tools and detect shadow deployments
These protections are critical wherever generative AI intersects with compliance, customer experience, or internal decision-making.
3. Vendors
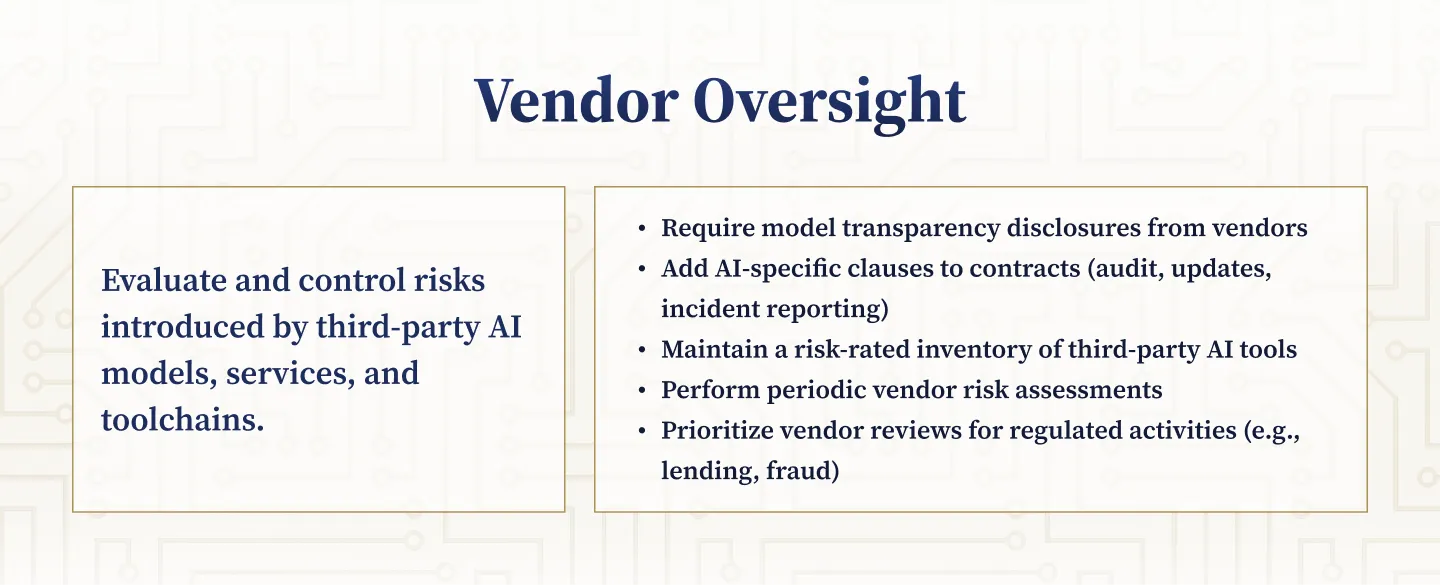
Understanding Risk from Third-Party AI Tools
Third-party AI vendors introduce significant risk when institutions depend on externally developed or opaque models. NIST recommends viewing vendor-hosted systems within the broader operational and regulatory context, ensuring their risks are evaluated alongside internal tools. Microsoft calls for transparency, explainability, and contract-level accountability when sourcing AI solutions.
The U.S. Department of the Treasury reinforces this view in its 2024 report, which highlights the disproportionate reliance of smaller institutions on third-party AI providers. Treasury notes the need for enhanced governance to address model opacity, inconsistent data usage terms, and limited visibility into training methodologies. Institutions are advised to strengthen due diligence and clarify vendor responsibilities for AI safety, bias mitigation, and regulatory readiness.
Vendor Oversight Practices That Align with Guidance
- Require detailed disclosures on model architecture, training data sources, and system limitations
- Include AI-specific language in contracts addressing data use, audit rights, incident response, and model updates
- Maintain an inventory of third-party AI tools, including their use case, risk rating, and compliance exposure
- Perform periodic vendor reviews, especially for models influencing credit decisions, fraud detection, or marketing
- Add generative AI evaluation questions to existing third-party risk frameworks
Institutions without dedicated vendor oversight structures for AI should integrate these practices into their broader sourcing and procurement protocols.
4. Data

Managing Data Inputs, Privacy, and Bias in AI Systems
Generative AI models rely on large, complex datasets for both training and inference. Poor data governance can introduce bias, reduce accuracy, and expose sensitive information. NIST emphasizes the importance of fairness, transparency, and privacy controls across the AI lifecycle. Microsoft recommends mapping data lineage, minimizing exposure, and enforcing strict access controls.
The U.S. Department of the Treasury identifies data privacy, quality, and representativeness as critical risk factors in its 2024 report. It highlights the risk of “data poisoning,” the challenge of ensuring compliance under fragmented state privacy laws, and the inconsistent application of GLBA standards across firms. Treasury recommends institutions adopt stronger data standards and clarify how data is sourced, shared, and used in both proprietary and third-party models.
Data Controls Critical for Safe and Compliant AI Use
- Assess training and operational data for demographic balance and potential bias
- Conduct privacy impact assessments for models using regulated or sensitive data
- Enforce anonymization, encryption, and role-based access controls
- Track data lineage across all AI systems to support auditability and regulatory reviews
- Continuously monitor data quality and refresh datasets to reflect current conditions
Institutions should ensure these practices align with applicable privacy laws (e.g., GLBA, CCPA) and internal data governance standards.
5. AI Model

Why Model Behavior Must Be Transparent and Measurable
The generative model itself represents one of the most significant risk surfaces. These systems produce content or decisions based on probabilistic logic that may be unexplainable to users or developers. NIST’s “Measure” and “Manage” functions stress the need for performance testing, explainability, and risk mitigation. Microsoft advises institutions to document model behavior and monitor for unintended outputs across the lifecycle.
In its 2024 report, the U.S. Department of the Treasury cites model opacity as a high-priority risk, particularly in use cases like credit underwriting and fraud detection. Treasury encourages financial firms to establish model governance processes that account for transparency, documentation, and measurable performance. It also notes that human review must remain part of any process involving high-impact decisions.
Model Governance Practices Based on Leading Frameworks
- Document each model’s intended function, inputs, training sources, outputs, and known limitations
- Use explainability tools like model cards or output audits to support stakeholder understanding
- Validate model performance before deployment and set a schedule for revalidation to detect drift
- Monitor output for biased, toxic, or non-compliant content, especially in customer-facing scenarios
- Apply additional controls to models used in regulated activities such as lending or reporting
These practices help mitigate regulatory and reputational risks while improving system trustworthiness and operational reliability.
6. Resiliency

Building Business Continuity Around AI System Dependencies
Generative AI systems, particularly those dependent on external APIs or open-ended model outputs, are prone to unpredictable failure. These may include latency issues, corrupted input handling, vendor outages, or hallucinated results. NIST calls for fail-safe mechanisms, redundancy, and fallback planning. Microsoft emphasizes disaster recovery readiness and model performance monitoring as part of secure AI development.
The U.S. Department of the Treasury, in its 2024 report, warns that excessive reliance on a limited number of third-party AI providers poses systemic risk—especially for smaller institutions without redundant infrastructure. Treasury recommends integrating AI failure scenarios into continuity planning, assigning clear operational ownership, and ensuring that institutions can maintain critical services during outages or model disruptions.
Key Practices for AI System Resilience
- Add AI-specific disruptions to business continuity and disaster recovery plans
- Establish manual fallback workflows for AI-supported operations
- Conduct failure mode analysis and stress testing for critical systems such as chatbots, underwriting, or fraud detection
- Monitor usage to detect instability or unsafe behavior, with defined triggers for suspension or rollback
- Train operational staff on emergency shutdown procedures and system bypass protocols
These safeguards ensure institutions can maintain core services and regulatory compliance even when AI components fail or behave unpredictably.
7. Compliance

Integrating AI Risk into Regulatory and Audit Programs
As generative AI becomes more embedded in financial workflows, its regulatory footprint expands. NIST recommends integrating AI risk into audit and governance frameworks. Microsoft emphasizes documentation, auditability, and system traceability to support compliance with legal and supervisory requirements.
The U.S. Department of the Treasury identifies compliance integration as a critical priority in its 2024 report. Treasury notes that financial institutions must proactively evaluate how AI affects adherence to consumer protection laws, data privacy regulations, and fair lending standards. It also highlights the need for consistent internal documentation and system-level visibility into AI-supported decisions.
Compliance Readiness Measures Drawn from Authoritative Sources
- Maintain a comprehensive inventory of AI use cases, mapped to relevant laws and guidance (e.g., GLBA, ECOA, CCPA, FFIEC)
- Review AI-generated outputs in compliance-sensitive processes such as onboarding, credit decisions, or fraud alerts
- Store testing results, validation records, vendor assessments, and approval logs in a reviewable format
- Include AI systems in internal audit scopes, risk assessments, and board-level oversight reporting
- Clearly document staff responsibilities, training requirements, and decision authority related to generative AI tools
Institutions without a formal AI compliance layer should begin by extending existing privacy, third-party risk, and IT governance programs to explicitly cover AI-enabled systems.
.avif)
THE GOLD STANDARD INCybersecurity and Regulatory Compliance
Key Outputs from a Generative AI Risk Assessment Process
What a Well-Documented Risk Program Should Include
Institutions seeking to align with guidance from NIST, Microsoft, and the U.S. Department of the Treasury should maintain documentation that supports both operational decision-making and regulatory readiness. Treasury’s 2024 report emphasizes the need for transparency, auditability, and cross-functional accountability in AI oversight. In combination with NIST’s lifecycle model and Microsoft’s governance playbooks, these sources outline the foundational outputs that institutions should be prepared to produce:
- A structured inventory of all AI systems in use or development, including ownership, intended function, risk classification, and update history
- A formal policy outlining AI approval processes, acceptable use cases, required documentation, and escalation protocols for system changes or incidents
- Comprehensive records for each AI model, detailing inputs, outputs, known limitations, validation results, and update schedules
- Evidence of controls across privacy, data lineage, vendor management, and system resilience, supported by versioned documentation
- An AI-specific risk register that is regularly maintained and integrated with the institution’s enterprise risk management framework
These deliverables demonstrate that generative AI is governed as part of the institution’s broader risk and compliance program—not as a siloed innovation project. They also serve as critical artifacts during audits, supervisory reviews, or incident response investigations.
Why Choose NETBankAudit for AI Risk Assessments
A Team of Experts Built for Financial Institutions
NETBankAudit was designed specifically to support the GLBA and FFIEC IT Regulatory Audit and Assessment needs of community financial institutions. Our firm’s structure, training, and methodology reflect this focus.
- We specialize in GLBA and FFIEC audits and assessments
- We help community financial institutions become and remain GLBA and FFIEC compliant
- Our team brings firsthand experience working within and alongside community financial institutions
More Than an Accounting or Technical Firm
NETBankAudit combines regulatory knowledge with deep technical expertise. Most firms lack this dual capability.
- Accounting firms generally do not have the technical and engineering expertise required for FFIEC-aligned IT audits
- Technical firms often do not have the regulatory or audit-specific expertise necessary for financial institution compliance
- We focus solely on assessments and remain independent from managed services, financial consulting, or product sales
Committed to Audit Independence
The FFIEC IT Audit Handbook highlights the risk of conflicts of interest when audit firms also provide consulting or financial reporting services. NETBankAudit avoids these conflicts by remaining fully independent.
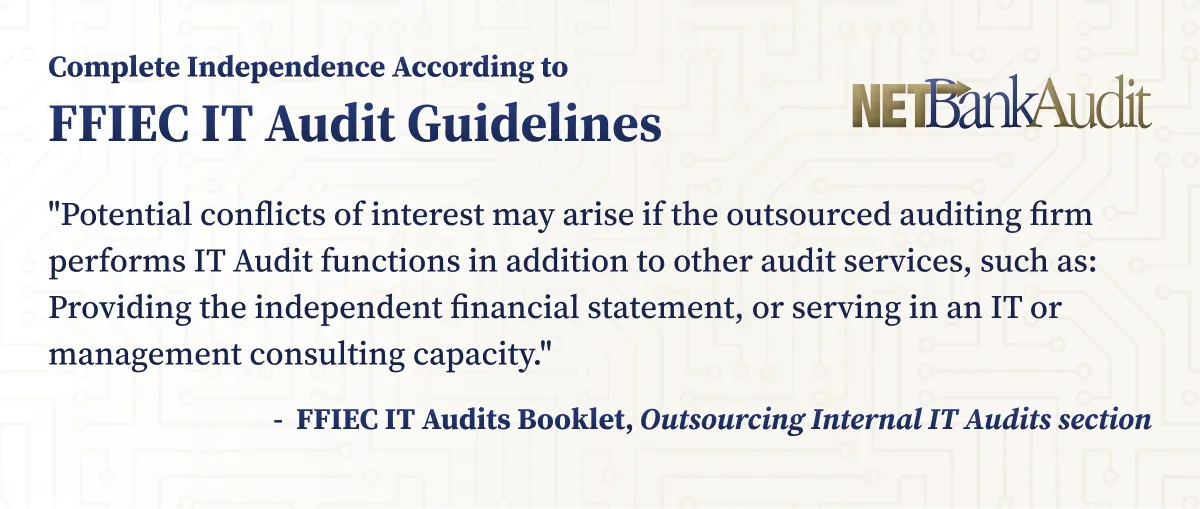
A Full-Time, Certified, and Trusted Team
Every member of our team is a direct NETBankAudit employee. We do not use subcontractors, and we maintain high standards for professional certification and integrity.
- All auditors and engineers are full-time employees
- Each employee holds applicable certifications such as CISSP and CISA
- Our team has real-world experience in financial institutions, security engineering, and regulatory audits
- All employees are subject to background checks for institutional trust and reliability
If your institution is evaluating generative AI risk, undergoing a cybersecurity audit, or preparing for FFIEC or GLBA compliance review, NETBankAudit provides the focused expertise and examiner-aligned approach you need.